Application questions, health interviews, motor vehicle records, attending physician statements, paramedical exams, blood and urine tests, and prescription histories are common elements of the life insurance underwriting assessment process. This standard look at mortality risk assessment utilizes a wide array of data – but is it really comprehensive?
As life insurers move toward accelerated or low friction underwriting, there is an increased interest in adopting emerging mortality risk prediction scores that are available almost instantaneously. One example of such a score interprets consumer credit attributes and public records. Another uses prescription (Rx) histories. Employing these scores effectively as predictors is no small task and requires unique expertise. Even when the mortality association of an individual underwriting data element is well established, it is still hard to determine the joint mortality risk association of multiple data elements and avoid double counting or improper weighting. This is a classic exercise in multivariate statistics.
For example, it is more likely that an applicant who is obese has a higher than normal blood pressure, higher cholesterol or has a family member with a history of heart attack. To assess the mortality risk accurately, an underwriter needs to measure the independent contribution to mortality risk from each element. This is called the exclusive protective value of each item of evidence.
The best way to derive the independent impact of multiple risk factors is to perform a longitudinal study in which all risk factors are measured at baseline and mortality outcome is followed longitudinally. Obviously, this kind of study is very difficult to conduct, if not impossible. Mortality is rare, so the study requires a large sample size and prolonged observation time. In addition, the vast universe of underwriting elements is hard to assemble. The challenges of the standard statistical method indicate the need for an alternative way to assess multivariate risk. Synthesis Analysis is just such an alternative method. To restate Synthesis Analysis in statistical terms: Assume we know the univariate relationship between risk factor 1 (X1) and mortality (outcome Y); assume we also know the univariate association of X2 versus Y, X3 versus Y… Xn versus Y. Synthesis Analysis allows us to construct the joint association of X1, X2…Xn versus Y, the desired multivariate association, if we know how X1, X2..Xn are correlated with each other.
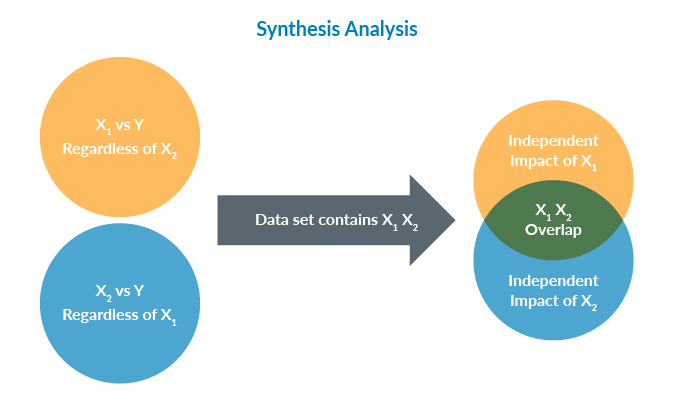
For example, suppose X1 is the consumer credit score, X2 is the Rx score and the outcome Y is mortality. To perform Synthesis Analysis, we need to know how the two scores are correlated to each other, which we can obtain relatively easily by performing a cross-sectional study of the two scores in a representative sample. This does not involve longitudinal observation or a large sample size because mortality is not needed. The Synthesis Analysis method is a practical solution to a common and intractable problem of multivariate analysis.
Gen Re holds an exclusive license to use Synthesis Analysis for insurance. Gen Re’s analytics team leverages Synthesis Analysis in our underwriting model development and research, and offers this expertise as a value-added service to our clients. Contact us if you are interested in applying Synthesis Analysis to help solve your specific underwriting problems.
Sources
- Hu G. et al., System and method for prediction disease onset. United States Patent Number 6,110,109.
- Hu G. et al., Adding multiple risk factors improves Framingham coronary heart disease risk scores. Vascular Health and Risk Management 2014: 10 557-62.
- Sheng E. and Hu G. et al., A new synthesis analysis method for building logistic regression prediction models. Statist Med 2014:33 2567-76.
- Zhou X. and Hu G. et al., Synthesis analysis of regression models with a continuous outcome. Statist Med 2009: 28 1620-35.
- Samsa G and Hu G. et al., Combining information from multiple data source to create multivariable risk models: Illustration and preliminary assessment of a new method. J of BioMed and BioTech 2005:2 113-23.
- Hu G. et al., Building prediction models for coronary heart disease by synthesizing multiple longitudinal research findings. Eur J Cardiovasc Prev Rehabil 2005: 12 459-64.